Insight Sustainability: ‘A just transition with farming community welfare at is core is the only approach that will work’ Niall O’Brolcháin on data, paludiculture and the future of farming
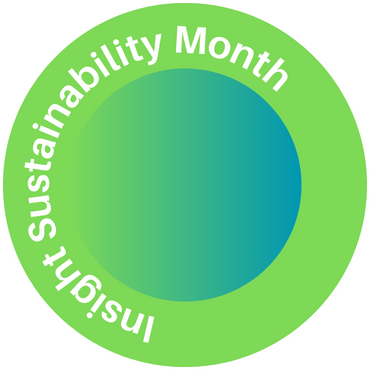
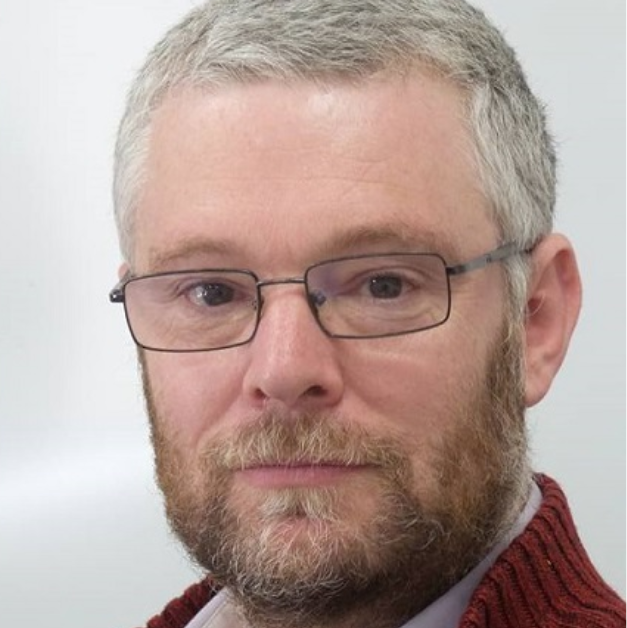
Engage with Insight - work with one of Europe's largest data science centres
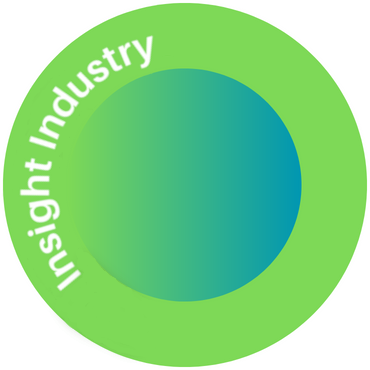
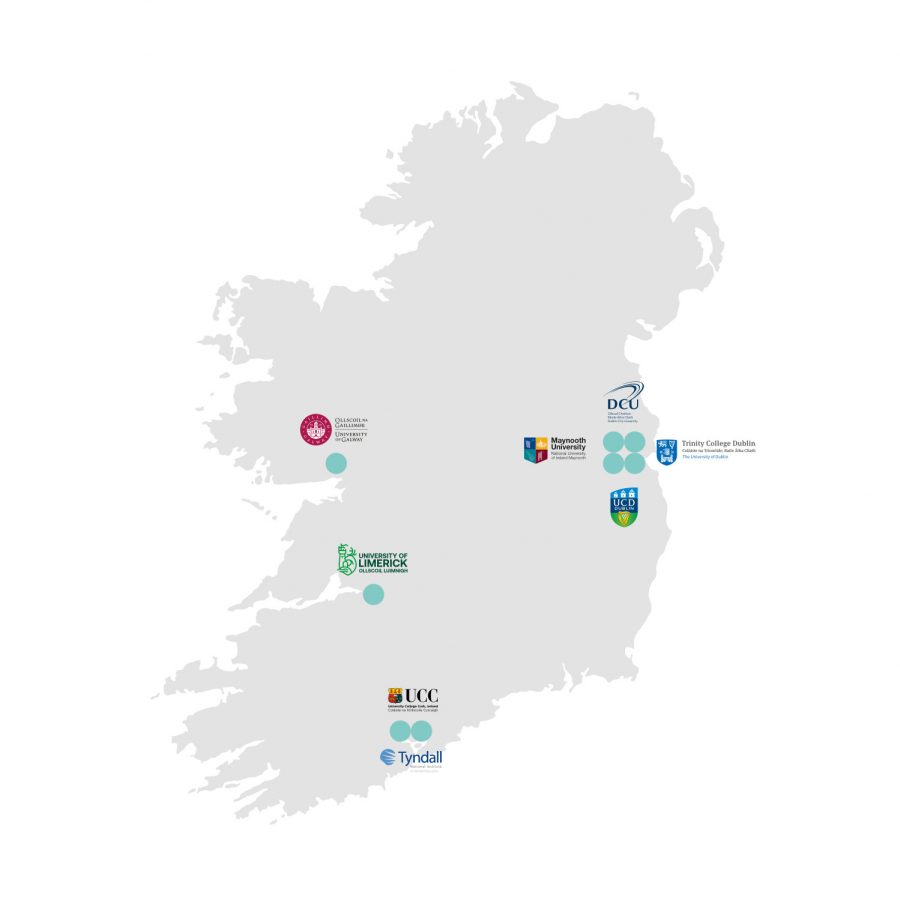
Insight is hiring! Check out our jobs page
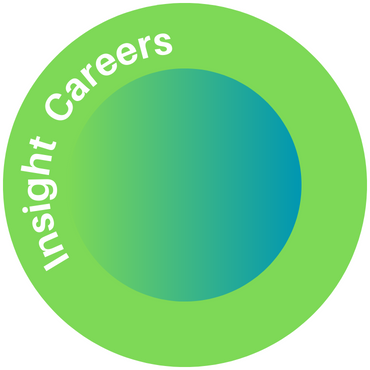
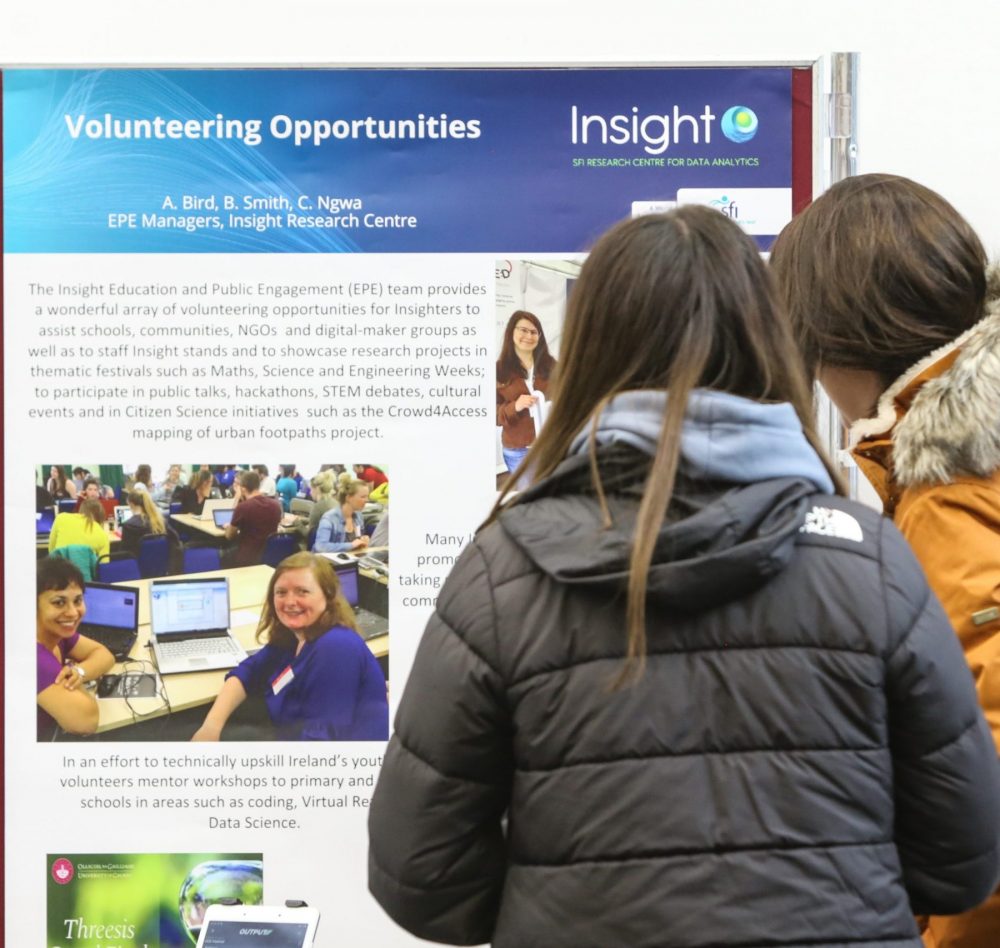
Latest News
Get our latest news in your inbox
Sign up for the Insight newsletter!
Insight in Numbers
0
+
Researchers
€ 0
+
Million in Funding
0
+
Industry Partners
0
Research Institutions